Tutorial 3 - scientific Python ecosystem 🐍: Xarray
(gridded data 🌐)#
In this tutorial we will to process and visualize raster data using PyGMT
’s integration with Xarray.
Note
This tutorial is part of the AGU24 annual meeting GMT/PyGMT pre-conference workshop (PREWS9) Mastering Geospatial Visualizations with GMT/PyGMT
Website: https://www.generic-mapping-tools.org/agu24workshop
Conference: https://agu.confex.com/agu/agu24/meetingapp.cgi/Session/226736
History
Author: Max Jones
Created: November-December 2024
Recommended versions: PyGMT v0.13.0 with GMT 6.5.0
Fee free to play around with these code examples 🚀. In case you found any kind of error, just report it by opening an issue or provide a fix via a pull request. Please use the GMT forum to ask questions.
0️⃣ What is Xarray?#
Xarray is an open source project and Python package for working with n-dimensional data using labeled dimensions and coordinates.
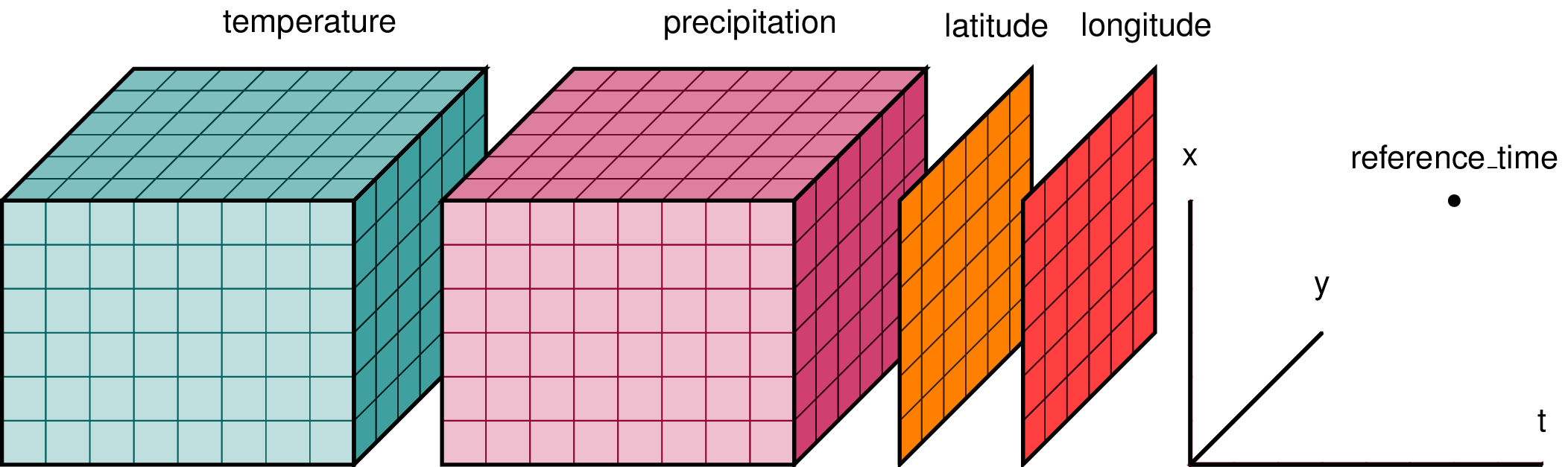
1️⃣ Why use Xarray with PyGMT#
Xarray provides a set of useful features for working with multi-dimensional data and unlocks even more functionality through its rich ecosystem. Some specific benefits of integrating Xarray and PyGMT include:
Simplifying the extension of PyGMT functionality to 3-D+ datasets
Access additional datasets, such as those located on the cloud
Use out-of-core computing with libraries like Dask
Interactively visualize by using Xarray, PyGMT, and dashboarding tools like Panel and Bokeh
Work with both raster and vector data in memory
2️⃣ Getting started#
First we’ll import all the libraries used throughout the tutorial.
import gcsfs
import pygmt
import xarray as xr
Let’s use a PyGMT function to explore an Xarray DataArray. We can use the pygmt.datasets.load_earth_relief
function to load one of GMT’s remote datasets. This will take longer the first time that it’s run because GMT downloads the data from the GMT server. We’ll specify the server in order to quicken download speeds to Washington, D.C., USA.
with pygmt.config(GMT_DATA_SERVER="NOAA"):
grid = pygmt.datasets.load_earth_relief(resolution="01d")
grid
gmtread [NOTICE]: Remote data courtesy of GMT data server NOAA [http://noaa.generic-mapping-tools.org]
gmtread [NOTICE]: SRTM15 Earth Relief v2.6 at 1x1 arc degrees reduced by Gaussian Cartesian filtering (314.5 km fullwidth) [Tozer et al., 2019].
gmtread [NOTICE]: -> Download grid file [112K]: earth_relief_01d_g.grd
<xarray.DataArray 'z' (lat: 181, lon: 361)> Size: 523kB array([[ 2865. , 2865. , 2865. , ..., 2865. , 2865. , 2865. ], [ 3088. , 3087.5, 3087. , ..., 3088.5, 3088. , 3088. ], [ 3100.5, 3100.5, 3101. , ..., 3101.5, 3101. , 3100.5], ..., [-3745.5, -3731. , -3723. , ..., -3734. , -3741.5, -3745.5], [-2939.5, -2945. , -2950.5, ..., -2895. , -2921. , -2939.5], [-3861. , -3861. , -3861. , ..., -3861. , -3861. , -3861. ]]) Coordinates: * lat (lat) float64 1kB -90.0 -89.0 -88.0 -87.0 ... 87.0 88.0 89.0 90.0 * lon (lon) float64 3kB -180.0 -179.0 -178.0 -177.0 ... 178.0 179.0 180.0 Attributes: Conventions: CF-1.7 title: SRTM15 Earth Relief v2.6 at 01 arc degree history: description: IGPP Earth relief long_name: elevation (m) units: meters vertical_datum: EGM96 horizontal_datum: WGS84
3️⃣ Plotting raster data#
We can pass this dataset to Figure.grdimage
to plot the dataset, specifying a projection and colormap. We use the Winkel Tripel projection (“R”) with a 12c width to show the whole world.
4️⃣ Processing raster data#
All of the PyGMT raster processing functions can accept and return Xarray DataArrays. As one example, we’ll use the grdgradient
function to create a hillshade raster. This will calculate the reflection of a light source projecting from west to east at an altitude of 30 degrees.
region = [-119.825, -119.4, 37.6, 37.825]
grid = pygmt.datasets.load_earth_relief(resolution="03s", region=region)
hillshade = pygmt.grdgradient(grid=grid, radiance=[270, 30])
hillshade
grdblend [NOTICE]: Remote data courtesy of GMT data server oceania [http://oceania.generic-mapping-tools.org]
grdblend [NOTICE]: Earth Relief at 3x3 arc seconds tiles provided by SRTMGL3 (land only) [NASA/USGS].
grdblend [NOTICE]: -> Download 1x1 degree grid tile (earth_relief_03s_g): N37W120
<xarray.DataArray 'z' (lat: 271, lon: 511)> Size: 1MB array([[-0.30839247, -0.37808514, -0.30799666, ..., 0.12039052, 0.20474193, -0.17929687], [-0.09469072, -0.3017121 , -0.31324536, ..., 0.0442035 , 0.06209851, -0.07466871], [-0.95000005, -0.95000005, -0.26423818, ..., 0.04321925, 0.03116445, 0.13370842], ..., [-0.44154865, -0.31297821, -0.20481637, ..., -0.1700097 , -0.22448991, -0.25389677], [-0.74398577, -0.27561006, -0.31929246, ..., -0.1227859 , -0.1983598 , -0.20921303], [-0.42644808, -0.35136998, -0.39577812, ..., -0.04772562, -0.19724692, -0.21158722]]) Coordinates: * lat (lat) float64 2kB 37.6 37.6 37.6 37.6 ... 37.82 37.82 37.82 37.83 * lon (lon) float64 4kB -119.8 -119.8 -119.8 ... -119.4 -119.4 -119.4 Attributes: Conventions: CF-1.7 title: Produced by grdgradient history: gmt grdgradient @GMTAPI@-S-I-G-M-G-N-000000 -E270/30 -G@GM... description: Lambertian radiance long_name: z actual_range: [ 508. 3533.]
Let’s plot the result using the same grdimage function shared earlier, this time using a custom colormap.
fig = pygmt.Figure()
pygmt.makecpt(cmap="SCM/grayC", series=[-1.5, 0.3, 0.01])
fig.grdimage(
grid=hillshade,
projection="M12c",
frame=["lSEt", "xa0.1", "ya0.1"],
cmap=True,
)
fig
5️⃣ Download data and load with Xarray#
Most times you’ll want to use your own data rather than the sample datasets. Here we show how to use the which
functionality to download a NetCDF file available via https.
# Download the dataset from the IRI Data Library
url = "https://iridl.ldeo.columbia.edu/SOURCES/.NOAA/.NODC/.WOA09/.Grid-1x1/.Annual/.temperature/.t_an/data.nc"
netcdf_file = pygmt.which(fname=url, download=True)
woa_temp = xr.open_dataset(netcdf_file).isel(time=0)
woa_temp
<xarray.Dataset> Size: 9MB Dimensions: (depth: 33, lat: 180, lon: 360) Coordinates: * depth (depth) float32 132B 0.0 10.0 20.0 30.0 ... 4.5e+03 5e+03 5.5e+03 * lat (lat) float32 720B -89.5 -88.5 -87.5 -86.5 ... 86.5 87.5 88.5 89.5 * lon (lon) float32 1kB 0.5 1.5 2.5 3.5 4.5 ... 356.5 357.5 358.5 359.5 time datetime64[ns] 8B 2008-01-01 Data variables: t_an (depth, lat, lon) float32 9MB ...
# Make a static plot of sea surface temperature
fig = pygmt.Figure()
fig.grdimage(grid=woa_temp.t_an.sel(depth=0), cmap="vik", projection="R15c", frame=True)
fig.show()
6️⃣ Open data directly from the cloud#
Xarray allows you to open data directly from cloud object storage. This can be useful to avoid downloading the entire dataset, since Xarray can subset to a specific section of interest before loading the data into memory.
Here we show this functionality based on Xarray’s tutorial. We’ll load a specific dataset from the Coupled Model Intercomparison Project Phase 6 (CMIP6), calculate the sea level change between 2015 and 2100, and plot the results using PyGMT.
First, let’s load CMIP6 data from Google Cloud Storage
fs = gcsfs.GCSFileSystem(token="anon")
store = fs.get_mapper(
"gs://cmip6/CMIP6/ScenarioMIP/NOAA-GFDL/GFDL-ESM4/ssp585/r1i1p1f1/Omon/zos/gr/v20180701/"
)
ds = xr.open_zarr(store=store, consolidated=True)
ds
<xarray.Dataset> Size: 268MB Dimensions: (lat: 180, bnds: 2, lon: 360, time: 1032) Coordinates: * lat (lat) float64 1kB -89.5 -88.5 -87.5 -86.5 ... 86.5 87.5 88.5 89.5 lat_bnds (lat, bnds) float64 3kB ... * lon (lon) float64 3kB 0.5 1.5 2.5 3.5 4.5 ... 356.5 357.5 358.5 359.5 lon_bnds (lon, bnds) float64 6kB ... * time (time) object 8kB 2015-01-16 12:00:00 ... 2100-12-16 12:00:00 time_bnds (time, bnds) object 17kB ... Dimensions without coordinates: bnds Data variables: zos (time, lat, lon) float32 267MB ... Attributes: (12/49) Conventions: CF-1.7 CMIP-6.0 UGRID-1.0 activity_id: ScenarioMIP branch_method: standard branch_time_in_child: 60225.0 branch_time_in_parent: 60225.0 comment: <null ref> ... ... tracking_id: hdl:21.14100/21c772eb-064e-4c75-8e16-da95bbfd29b7... variable_id: zos variant_info: N/A variant_label: r1i1p1f1 netcdf_tracking_ids: hdl:21.14100/21c772eb-064e-4c75-8e16-da95bbfd29b7... version_id: v20180701
Calculate the different in sea level between a date in 2100 and 2015
zos_2015jan = ds.zos.sel(time="2015-01-16").squeeze()
zos_2100dec = ds.zos.sel(time="2100-12-16").squeeze()
sealevelchange = zos_2100dec - zos_2015jan
Make a plot of the difference in sea level